A Novel Benchmark for Domain Generalisation in Humanitarian and Climate Applications
The Challenge of Real-World Deployment
Object detectors have achieved remarkable performance in controlled environments, but they often fail when deployed in real-world scenarios. Why? Most models are designed under the independent and identically distributed (i.i.d.) assumption—trained and evaluated on data from the same distribution.
However, real-world applications face significant distribution shifts between source (training) and target (deployment) data, resulting in substantial performance degradation. This gap is particularly problematic in high-stakes domains like humanitarian response and climate change monitoring.
Introducing RWDS
To address this critical gap, we are proud to announce the release of Real-World Distribution Shifts (RWDS), a suite of three novel benchmarking datasets specifically designed for assessing object detection under realistic Domain Generalisation (DG) scenarios.
RWDS focuses on humanitarian and climate change applications, enabling researchers to investigate domain shifts across:
- Climate zones
- Various disaster types
- Different geographic regions
To our knowledge, these are the first DG benchmarking datasets tailored for object detection in real-world, high-impact contexts.
Why RWDS Matters
Domain Generalisation (DG) aims to bridge the gap between controlled lab environments and unpredictable real-world deployments by enabling models to generalize to Out-Of-Distribution (OOD) data—without requiring access to target distributions during training.
By providing standardized benchmarks for evaluating robustness against spatial domain shifts, RWDS will accelerate research in creating more reliable and deployable computer vision systems for critical applications.
Applications
RWDS has immediate applications in:
- Robust computer vision research
- Disaster damage assessment
- Climate change adaptation
- Humanitarian applications
Datasets
RWDS-CZ: Evaluating Object Detection in a Changing Climate
As climate change continues to impact natural resources, weather patterns, and ecosystems, there is a growing need for computer vision models that can adapt to these evolving conditions. In particular, object detection must remain robust across diverse and shifting climate zones.
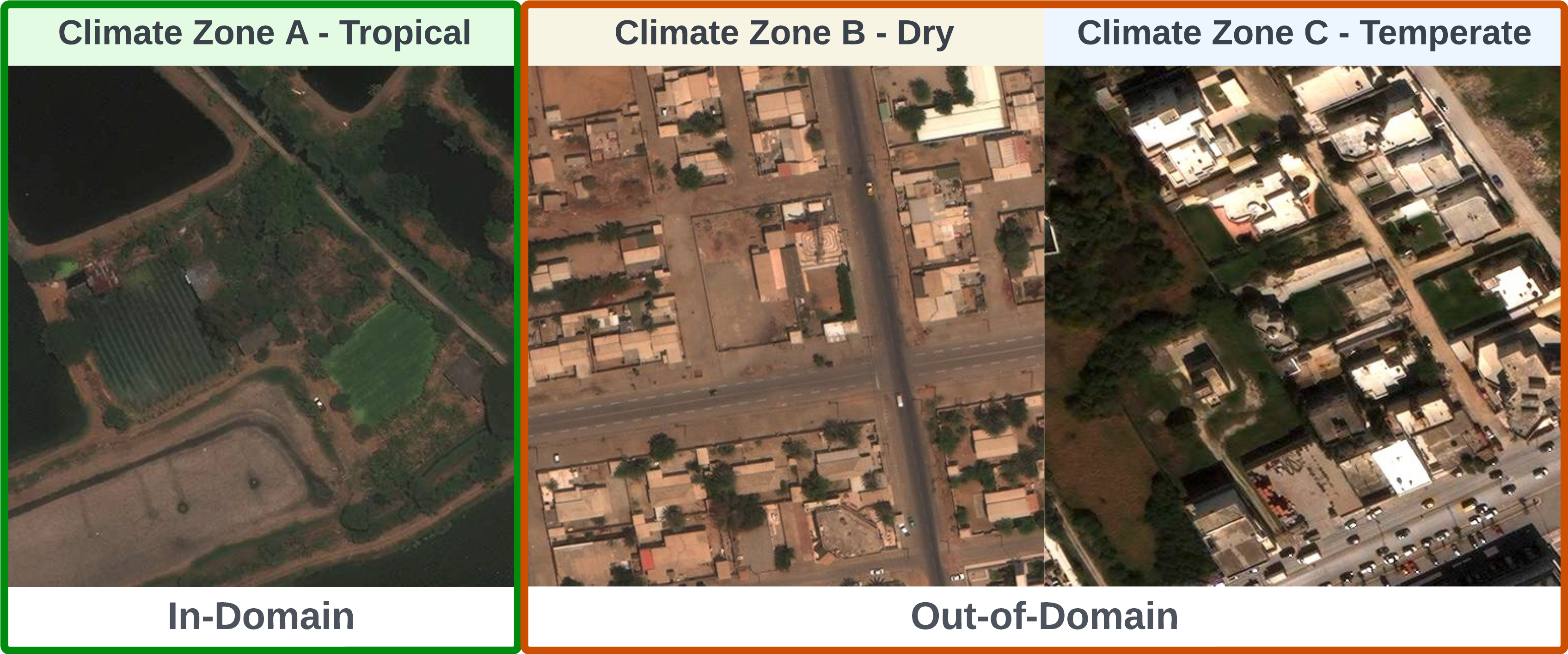
To address this, we introduce RWDS across Climate Zones (RWDS-CZ)—a challenging dataset designed to evaluate model resilience and generalisability based on Köppen’s climate classification. By testing object detection across varied environments, RWDS-CZ provides a valuable benchmark for the research community, enabling the development of more robust and adaptable models to meet real-world challenges.
RWDS-FR: Evaluating Object Detection Across Flooded Regions
Floods are among the most devastating natural disasters, often affecting regions with vastly different geographical and socio-economic conditions. To evaluate how well object detection models generalise across such variations, we introduce RWDS across Flooded Regions (RWDS-FR).
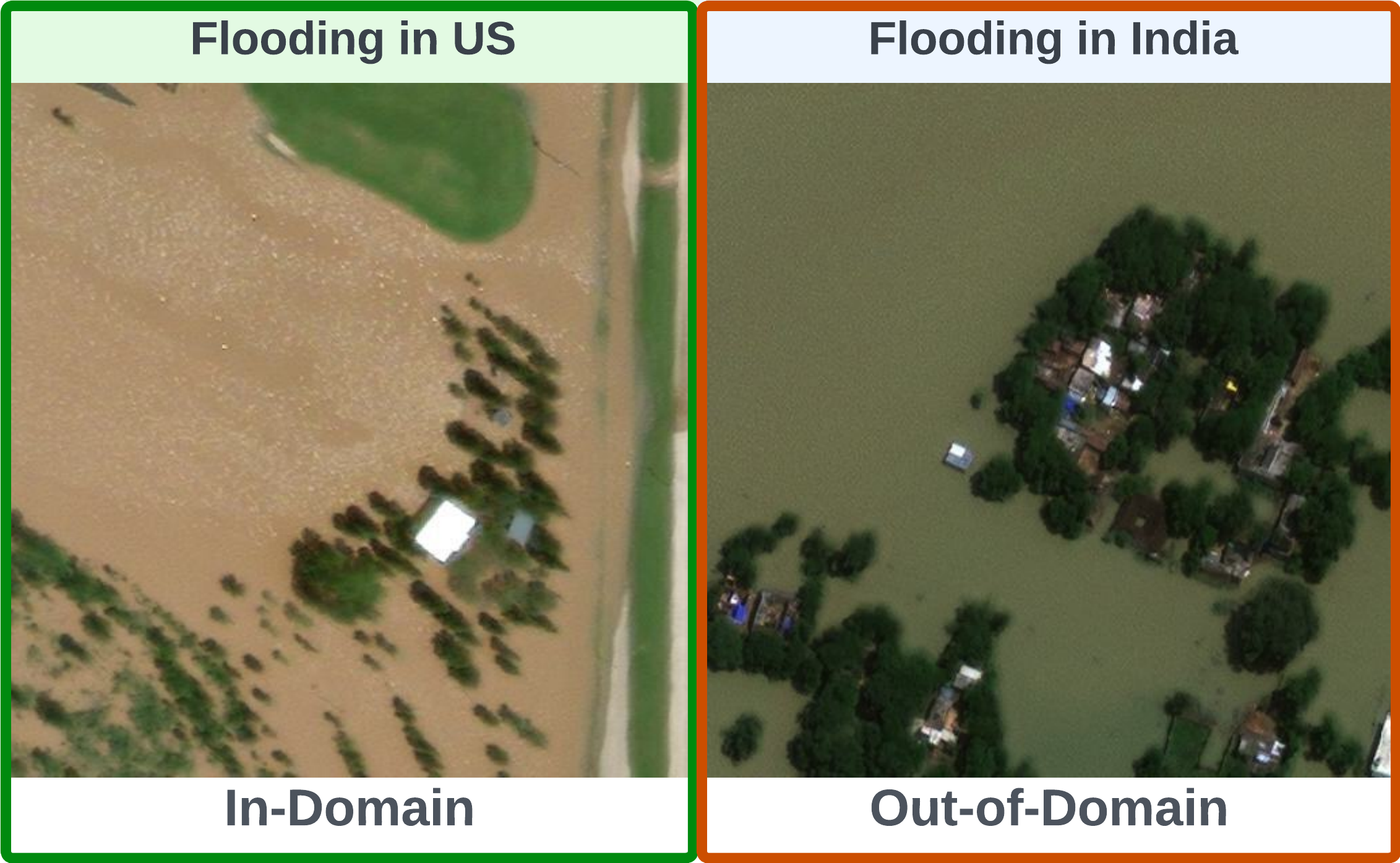
This dataset compares flood events in the United States and India, examining how state-of-the-art (SOTA) object detectors perform when applied to satellite imagery from these distinct locations. By testing model robustness in this setting, RWDS-FR aims to advance the development of AI systems capable of adapting to diverse flood scenarios without requiring extensive re-training.
RWDS-HE: Understanding Model Robustness Across Hurricanes
Hurricanes vary significantly in impact, even within the same geographical region. To assess how object detection models handle these differences, we introduce RWDS across Hurricane Events (RWDS-HE).
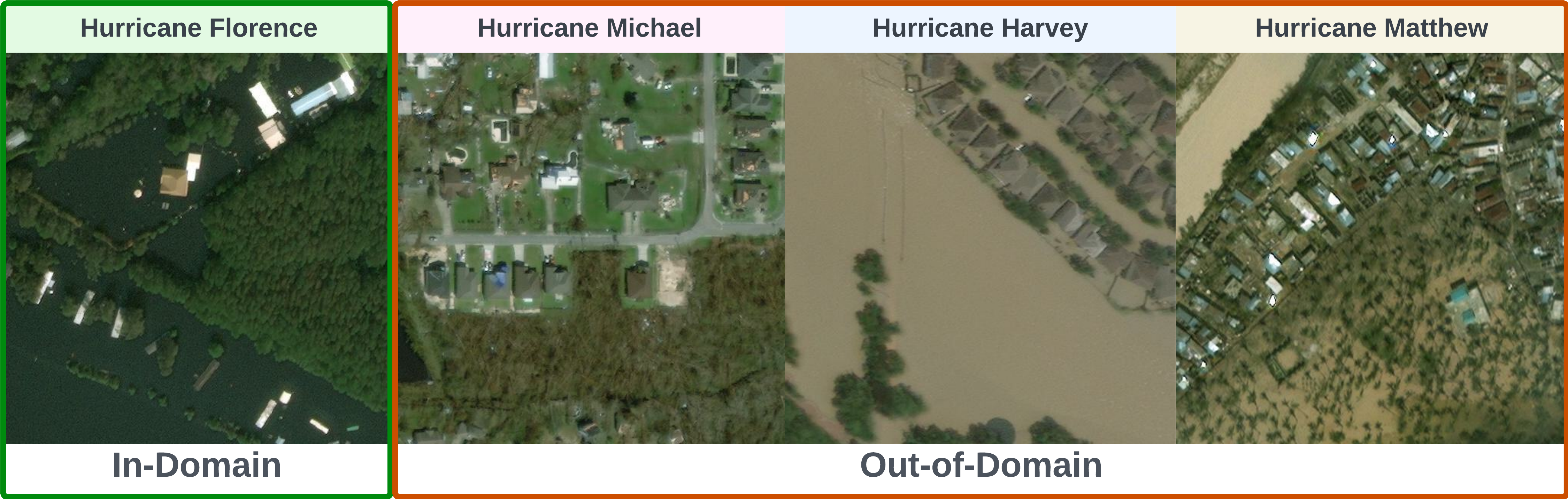
This dataset focuses on multiple hurricane events across North America, evaluating how well models generalise to different storms. Instead of studying geographical shifts, RWDS-HE explores variations in damage patterns, intensity, and affected infrastructure. RWDS-HE provides a benchmark for improving AI-driven disaster assessment tools, ensuring more reliable damage detection across diverse hurricane events.
Access the Resources
Citation
If you use RWDS in your research, please cite our paper:
@inproceedings{rwds_2025,
title = {{Benchmarking} {Object} {Detectors} under {Real}-{World} {Distribution} {Shifts} in {Satellite} {Imagery}},
author = {Al-Emadi, Sara and Ofli, Ferda and Yang, Yin},
booktitle = {IEEE Conference on Computer Vision and Pattern Recognition (CVPR)},
year = {2025}
}
Contact
For questions or collaboration opportunities, please contact: salemadi@hbku.edu.qa